The Project
Decisions based on data-driven insights can be vital and have a phenomenal impact on the environment, society, and business. Many critical domains such as crisis management, predictive maintenance, mobility, public safety,and cyber-security become increasingly disrupted by new means to harness the extreme proliferation of data for effective decision making. Generating data-driven insights that can be used and trusted by decision-makers is, however, still far from trivial. On the one hand, (big) data analytics solutions need to cope with data of extreme scale, low quality, different modalities, and different owners. On the other hand, complex data analytics comprising machine learning and simulation tasks need to provide outcomes that are both accurate, precise, and fit for purpose. On top of these needs, insights are not used in decisions if they cannot be trusted. Therefore, increased trustworthiness in data-driven insights is pivotal in the adoption of data-driven decision making, especially in life-critical domains.
In response to this dire need for decision making based on accurate, precise, fit-for-purpose,and trustworthy data-driven insights, ExtremeXP proposes a new paradigm for data analytics, which we call experimentation-driven analytics. The main contribution is that it puts the end user at the center of complex analytics processes from data discovery to novel interactions, proposing a human-in-the-loop, experimentation approach for gaining knowledge and making decisions from data with varying and extreme characteristics. This way, it gradually builds up knowledge to associate users to complex analytics workflows that meet their needs.
ExtremeXP will integrate interactive visualization and explainability techniques to increase the trustworthiness of not only the outcomes but also of the process to reach such outcomes. Towards the latter, it is important to transparently and immutably persist any access control decisions regarding datasets used for deriving valuable insights and highlight their characteristics and therefore potential value in terms of the data analysis workflow. The provided framework automates the process of running complex analytics as part of experiments and of building up the knowledge base for user-experience-driven analytics, reducing the complexity associated with manual tuning of complex analytics.
Our approach in implementing the ExtremeXP concept is to provide a modular framework which orchestrates different subsystems. At the core lies the Experimentation Engine containing the core artifacts of the framework that are related to modelling and planning experiments, enhancing experiment descriptions with context information, scheduling the execution of complex analytics workflows in the available infrastructure, and monitoring its execution as well as its properties (using both system metrics and user metrics). The experimentation engine will be offered as a service.
It is composed of four core artifacts:
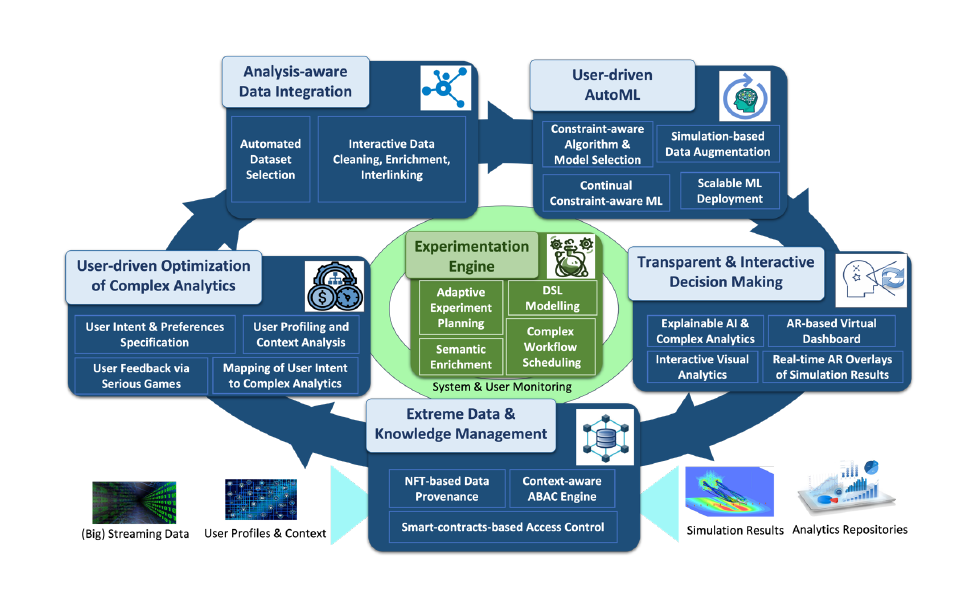
User-driven AutoML
contains the artifacts that consolidate the novel ML research of ExtremeXP and include simulation-based data augmentation for ML, constraint-aware ML algorithms, algorithms for model selection based on user preferences and constraints, continual learning of model selection strategies and optimal deployment of ML pipelines in heterogeneous environments.
User-driven Optimization of Complex Analytics
encompasses the frontend for capturing user intents, requirements, and constraints, as well as user feedback via gamification. It also contains artifacts used for mapping intents to variants of complex analytics processes that can run in the backend, as well as for using the rich information of the users and their context to create user profiles that can be further exploited by the ExtremeXP framework for personalizing the experience of end users, anticipating their intents and preferences
Transparent & Interactive Decision Making
provides the artifacts responsible for enhancing the users’ trust and experience via providing explanations on the choice and configuration of an ML/data analytics or simulation method used by the framework. Interactive visualization and AR technologies are used both in enhancing explanations and result description and in offering intuitive and fast ways for the users to interact with the experimentation engine.
Analysis-aware Data Integration
deals with data-processing related challenges and provides novel solutions for automatically selecting among alternative datasets, dealing with data quality issues such as missing, incomplete, wrong, and duplicate data points, in user-driven reconfigurable workflows.
Extreme Data & Knowledge Management
provides capabilities for secure and distributed management of datasets and experimentation-based knowledge assets and learning outcomes. The subsystem will support the complete life cycle of knowledge asset management, i.e., knowledge elicitation, representation, persistence, organisation and sharing, and will enable users to access securely relevant know-how to design, perform and evaluate advanced data analytics. Therefore, it will introduce the holistic management of data and knowledge required for deriving different complex analytics variants, along with their extreme data analysis outcomes, in a secure and efficient manner that copes with the heterogeneity and distribution of all data artefacts involved.